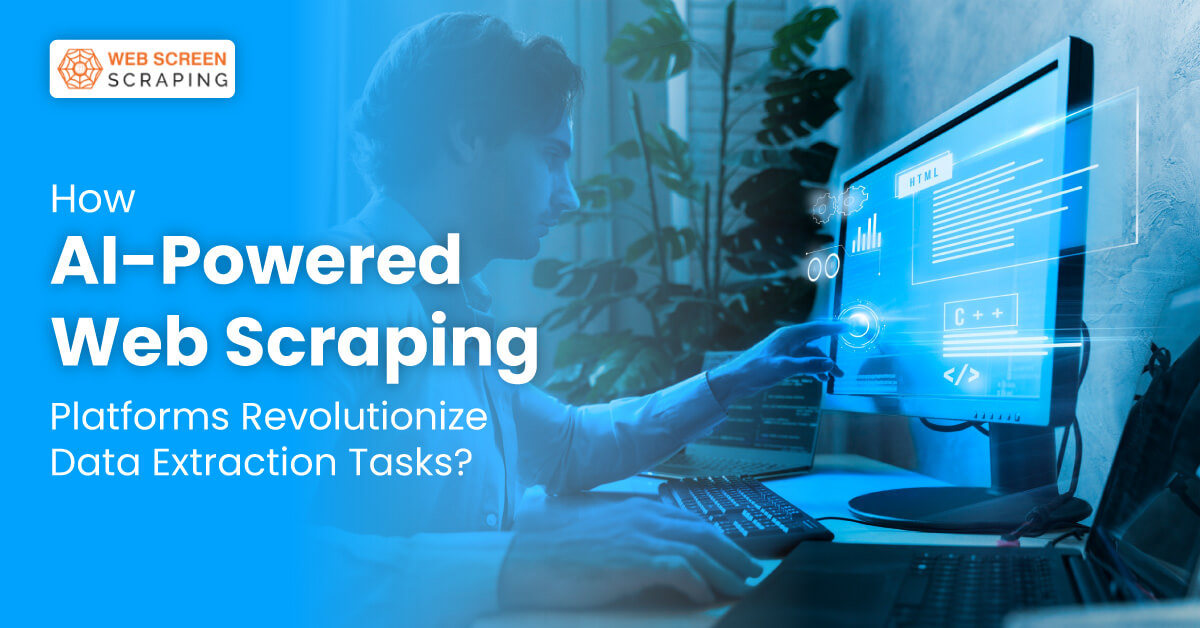
In the digital transformation era, “Data is the New Oil.” Data insights have become an inevitable asset as businesses rely on data-driven decisions. Companies adopting data scraping solutions or services to gather vital information from various online data sources. The collected information is useful for research, competitor analysis, identifying market trends, revealing consumer needs, finding new business opportunities, and more.
Web scraping services and tools help gather a large volume of required data anytime, according to business needs. Traditional web scraping methods can be error-prone as they are done with manual coding, specifically while extracting large chunks of data. Artificial intelligence plays a significant role in improving the process of data scraping along with improving data quality and accuracy. AI-powered solutions are changing how data is collected by quickly completing the process even with the huge data requirement.
Today, we will learn how AI is helping the data scraping industry by simplifying the complex process.
What is AI Web Scraping?
AI-based web scraping is the process of extracting data in an automated way from various sources using advanced technologies like NLP, ML, and computer vision. Compared to traditional web scraping, AI-based data extraction is done with minimal human intervention and scripts.
AI-powered tools extract and deliver accurate and quality data, which is crucial for companies to make critical business decisions, understand customer requirements, track competitors, identify market trends, stay ahead of the competition, and more.
The Rise of AI in Web Data Scraping
With the evolution of digital transformation, AI has become the first technology solution for many industries. Artificial Intelligence-based solutions help to complete processes quickly and effectively.
AI Data Scrapers know the extraction patterns and adjust with the dynamic web structures without any human intervention. Advanced ML and NLP algorithms enhance the capabilities of extracting data with utmost precision in a well-structured format.
Convolutional Neural Networks improvise image data extraction by helping AI to identify visual contents across the internet. Moreover, the RL (Reinforcement Learning) model assists in making optimal scraping activities better.
A combination of AI algorithms and scraping tools results in the following:
- Perfect data classification and analysis
- Better adaptability and process with dynamic web structures
- Minimal human interventions
- Large dataset extraction can be managed very easily
How is AI Simplifying Complex Data Extraction?
With the rise of AI-enabled platforms, the data extraction process also become automated and simplified. These advanced tech systems help businesses quickly gather large amounts of data and ensure a smooth analysis process.
Enhancing Data Quality
Maintaining data quality is one of the major challenges with traditional web scraping methods.
Most of the time, extracted content remains inconsistent, full of error, and sometimes covers irrelevant data that may represent the wrong information and affect critical business decisions.
Here, AI comes to the rescue with advanced ML algorithms capable of gathering unique data patterns, removing unwanted data, and minimizing errors while collecting critical data insights. For example, NLP helps to correct the data by mitigating the punctuations, stopping characters, and other words that are not necessary in the dataset. Computer vision-based AI models collect precise graphical details from data sources such as images, videos, or documents uploaded on web platforms.
Managing dynamic web platforms is very important to get the utmost data quality. AI-enabled solutions can handle frequent changes in the webpage structure. AI data scraping tools swiftly adjust to the new design due to constant training and enhancement. Nowadays, improved learning neural networks can identify compound structures of data source platforms and regulate the data collection process without or with minimal human intervention.
Restructuring Data Scraping Procedures
In outdated web scraping methods, explicit rules must be set for every targeted domain, which can take ample time to set up and is full of errors. Artificial Intelligence utilizes ML algorithms to systemize the rule-building process, which helps to enhance scraping proficiency and minimize manual methods. AI-based data scrapers set the priority of the data collection process based on data relevancy, timeline, or priority to ensure that the end user gets exact information without pouring much effort into the vast raw dataset.
Achieving continuous scalability over numerous platforms at a time is possible by incorporating AI skills into the data scraping tools. Enhanced AI algorithms can crawl and analyze numerous data sources simultaneously, check similarities, and deploy the finest data extraction methods similar to targeted platforms or pages. This results in increased productivity and minimum resource supplies.
Controlled Learning for Pattern Identification
To recognize data patterns effectively during web scraping, managed learning is essential. Here, the AI model is getting trained with categorized data sources. This process involves the collection of applicable pages and applying labels on the data that define particular data elements such as product name, price, details, ratings, reviews, and more.
The model can be trained with the features available on the targeted pages, such as texts, HTML code, CSS, visuals, and more. ML algorithms are the subject of choice per task and complex data extraction situations.
Trained models perform accurate predictions on the web pages to find out and scrape data information. Once data is extracted, later processes ensure the acquired data is accurate and useful.
Data Filtering
Artificial Intelligence identifies the difference between relevant and irrelevant content. While scraping data from web pages, AI scrapers leave the data related to ads, menus, and footers to ensure that only useful information is gathered. Data filtration is achieved by cutting-edge classification and advanced techniques. This process saves ample time related to data gathering and filtering compared to traditional web scraping.
Managing Complex Data Structures
AI web scraping handles complex data structures more efficiently than old data scraping methods. Advanced algorithms and technologies find and sort data in a classy manner from any complex source structure. With AI, gathering data from dynamic web and mobile applications quickly becomes easy.
Better Scalability and Automation
AI-based web scraping tools are easily scalable, which makes them a perfect choice for large-scale data extraction. Millions of data records from thousands of web platforms can be collected quickly without compromising accuracy. Automation is the key benefit of AI adoption, as the data scraping tasks can be scheduled in advance to retrieve data at regular intervals without monitoring the process. Automated data extraction is inevitable for businesses that depend on real-time data such as finance, eCommerce, news, and more.
Data Cleaning and Better Data Structure
Data collected with outdated methods can be messy, have wrong information, broken links, and bad structure format. AI tools are experts when it comes to data cleaning and structuring. NLP and ML techniques clear the unwanted information and arrange the data in a well-structured format before delivery. This process covers removing duplicate data, correcting formatting errors, translating texts into the desired language, and more. For example, while extracting data from multiple eCommerce platforms, AI-based tools normalize product names, details, categories, prices, ratings, and more to make the data consistent and available for accurate analysis.
Taking Care of Anti-Scraping Measures
AI-enabled scraping tools are trained to manage anti-scraping measures with ease. While gathering the data in an ethical manner to optimize your products and services, AI tools can pass the captcha and other anti-scraping techniques implied by the web platforms without getting tracked.
Intelligent Data Recognition
Finding the right data on targeted web pages is one of the major challenges in the web scraping process, particularly when dynamic layout is involved. AI scraping tools resolve this issue with the help of intelligent data recognition. AI scrapers and APIs utilize ML algorithms to find and collect data with precise relevancy from web pages. Advanced scraping tools can identify patterns, understand content, and extract structured and unstructured data.
Proxy Rotation and Server Management
While scraping data from any web platform, it Is important to hide your real IP to avoid getting blocked from the targeted domain. This can be achieved with the proxy rotation. Managing and rotating proxies with manual efforts is very difficult while scraping large volumes of data. AI scraping tools are trained enough to manage and switch the proxies in an automated way.
Effectively managing the server is also very important while performing web scraping to handle a high volume of data requests and extraction processes. AI data scraping platforms help manage server infrastructure better for smooth and consistent data collection.
The Future of AI in Web Scraping
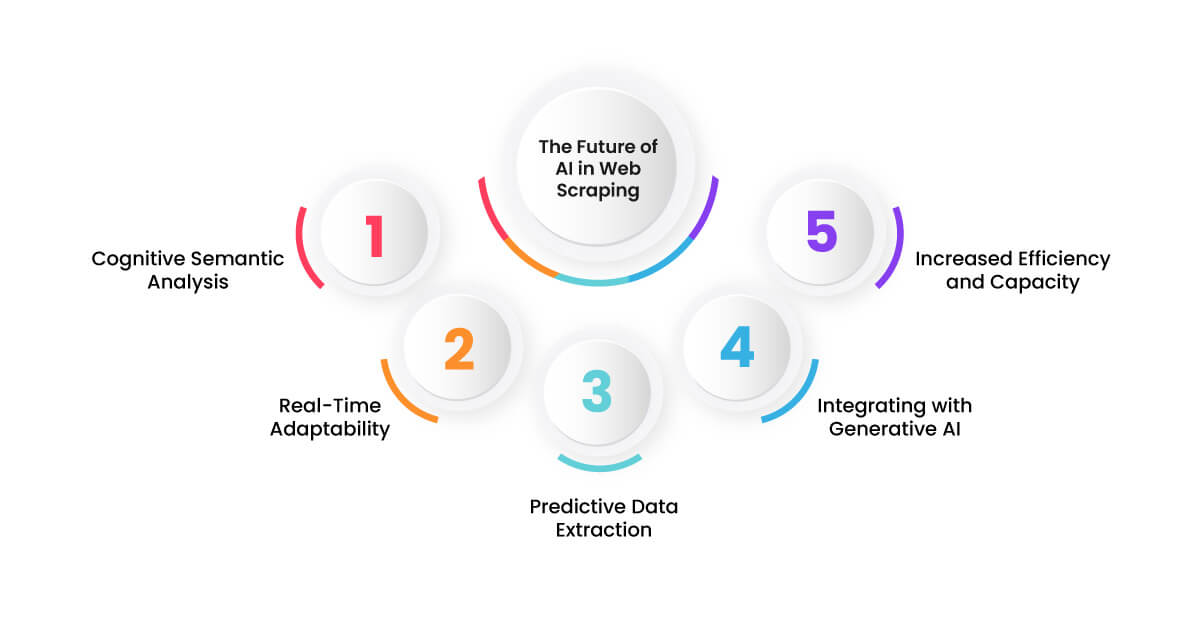
The field of web scraping is evolving rapidly, and AI technologies are at the forefront of this transformation. Here’s how the future of AI will shape and enhance web scraping capabilities:
Cognitive Semantic Analysis
AI will continue to use natural language processing (NLP) for better analysis of content about the context in which it was published for better results on data mining. For example, sentiment analysis can be derived from reviews or product descriptions. Sophisticated analysis will deconstruct the specific entities, including the name, price and date, regardless of the other information that may accompany it.
Real-Time Adaptability
It will help AI systems respond more effectively to website design changes, including alterations in HTML layouts or content management systems that are more frequent than with print media, for example. Machine learning models will learn and simulate various possible changes on the website to maintain the continuity of the data extraction process.
Predictive Data Extraction
AI is expected to advance beyond merely scraping static data and predict trends based on scraped data over time, such as tracking the prices in e-business or analyzing shifts in job offers to anticipate future demand in the field. Subsequent platforms could create real-time notifications for changes in important status, like a new low product price or a new competitor update.
Integrating with Generative AI
Some non-commercial use of generative AI models like GPT includes ‘teaching’ the model to develop a unique scraping script or API relevant to the users’ requirements. AI could summarize insights, and what is seen is precisely what has been highlighted.
Increased Efficiency and Capacity
Integrating AI with RPA ( Robotic process automation) will result in end-to-end solutions for scraping, cleansing, and integrating data into various processes. Cloud-based platforms will continue popularizing AI solutions, allowing for the scraping and processing of massive amounts of data without requiring installations.
Conclusion
AI web scraping tools and platforms revolutionized data extraction by making it simpler, faster, and more efficient. AI tools allow for gathering vast amounts of valuable data with intelligent data identification, automated processes, and adaptability to dynamic website structure changes. Whether you are a business analyst, market researcher, or developer, AI-based data scraping tools deliver robust and scalable solutions to manage complex data extraction. Get accurate, error-free, and well-structured data quickly with Web Screen Scraping to unlock the potential of the key data insights for better business decision-making.