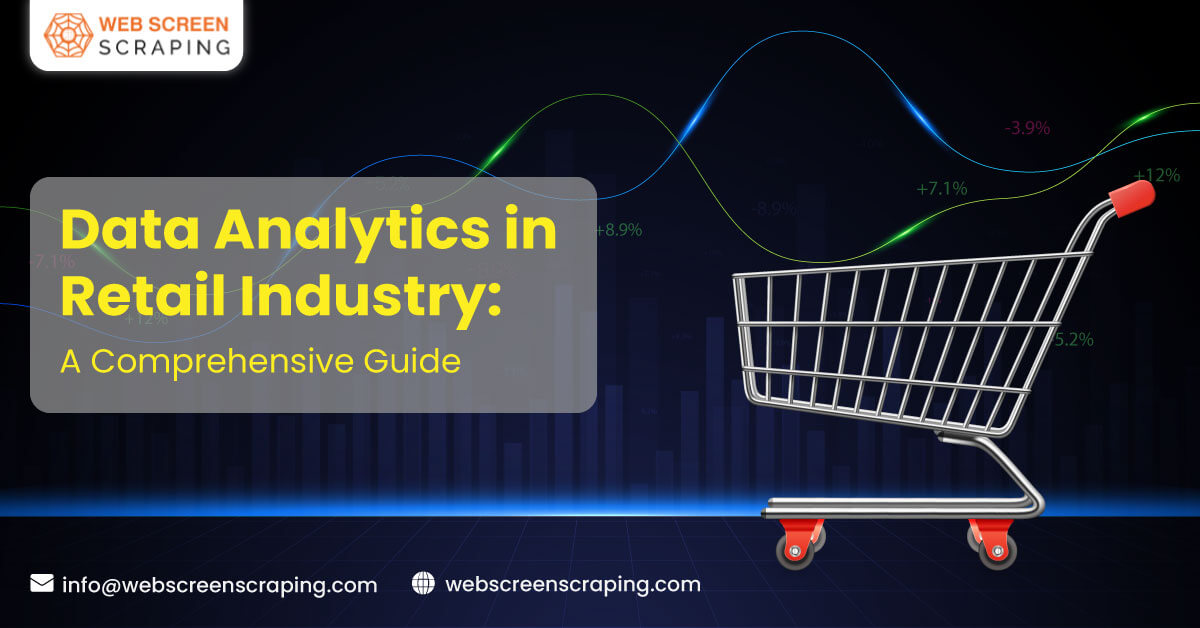
The retail industry is very competitive these days. Businesses use data analytics for retail to make better decisions, understand customer behavior, and improve their operations. In this guide, we will explore the importance of data analysis in the retail industry, how it's changing, and how retailers can use it to succeed.
What is Data Analytics in Retail?
Data analytics in retail means gathering, organizing, and analyzing the information stores create. This information comes from different sources, such as sales, customer interactions, stock levels, etc. The aim is to find repeated actions, tendencies, and valuable information that can assist stores in making better choices.
Data analytics for retail is used increasingly by big retail companies worldwide to track popular products, forecast sales and demand, and optimize product placements and offers. It also helps determine which customers like certain products based on what they have bought before and creates specific ways to advertise to them.
Why is Data Analytics Important in Retail?
The retail industry gathers much information every day. This information comes from various sources, such as online sales, in-store purchases, customer interactions, and supply chain logistics. If we study this information carefully, we can discover essential insights that help us make good decisions. Data analysis in retail industry plays a crucial role for several reasons:
Understand Customer Behavior
Analyzing customer information can help retailers understand shopping habits, preferences, and trends. That allows stores to improve their ads, make shopping fun for customers, and sell more things.
Optimizing Inventory Management
Retail Big Data analysis helps retailers manage their products better. It can predict customer demand and popular items so stores stay supplied and have enough extra stuff.
Enhancing Pricing Strategies
Data analytics for retail help retailers determine the best prices by examining what other stores are charging, what customers want, and how things change throughout the year. That ensures the prices are reasonable enough to compete with other stores and make the most money.
Improving Store Operations
By analyzing retail location data, retailers can figure out the best places to open new stores, organize the inside of the stores better, and make operations run smoother to work better.
How to Implement Data Analytics Solutions for Retail?
When implementing data analytics solutions for retail, there are several steps to follow:
- First, gather important information from different sources, such as sales records, customer opinions, and social media activity.
- After getting the information, it needs to be saved in a way that is easy to study. Retailers often use retail store datasets, retail product datasets, and other retail datasets for this purpose.
- The next step is to study the data using different tools and methods, such as descriptive, predictive, prescriptive, and real-time analytics to gain insights from the data.
- Finally, stores use the ideas they get from studying the information to make smart business choices, like managing their products well and beginning special marketing efforts.
How Retailers Use Data Analytics?
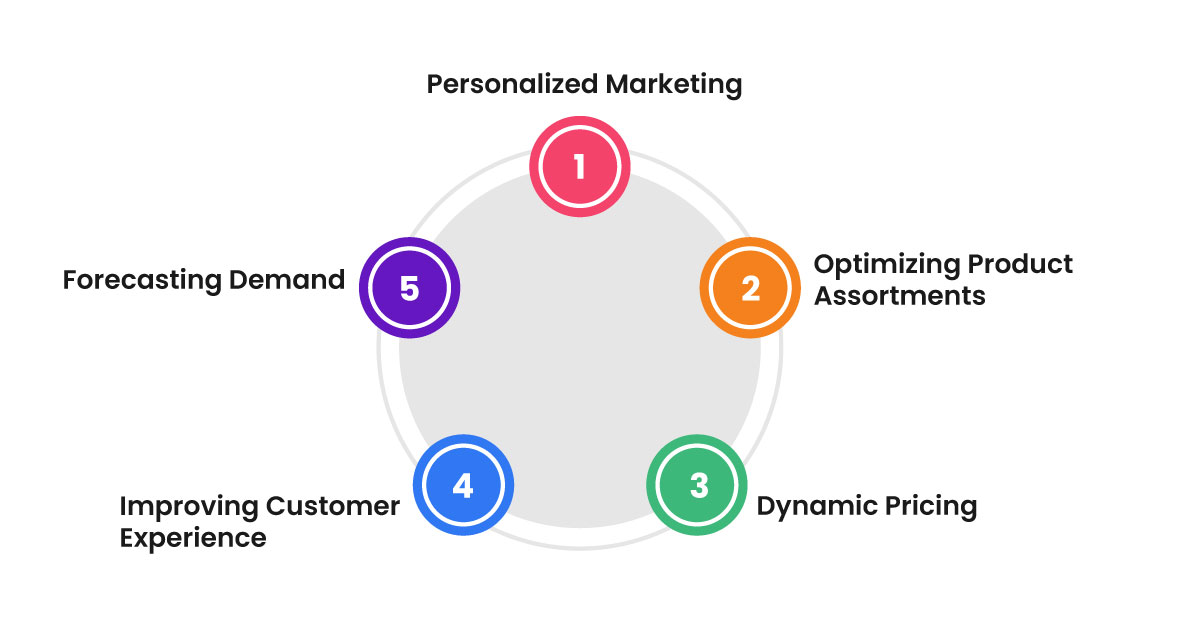
Data Analytics Retail mainly improves operations and customer experience and increases sales. Here are some main uses:
Personalized Marketing
Data analytics for retail helps retailers make unique marketing plans by analyzing customer information. By knowing what each customer likes, retailers can send customized marketing messages, suggest products, and offer special deals, which helps increase customers' interest and sales.
Optimizing Product Assortments
Retailers can use sales and inventory data to determine popular retail product datasets. That helps them stock more of what customers want and reduce items that don't sell, resulting in increased sales and better inventory management.
Dynamic Pricing
Dynamic pricing is a method where prices change quickly depending on how much people want something, what other stores charge, and how the market is doing. Data analysis in the retail industry is essential for dynamic pricing because it helps retailers determine the best prices to charge at different times. This method allows stores to make as much money as possible and stay competitive.
Improving Customer Experience
Data analytics for retail can help retailers understand what their customers like to buy and how they like to shop. By looking at things like customer feedback and what people have purchased before, retailers can find ways to improve their customers' experiences. That can lead to increased customer loyalty and repeat business.
Forecasting Demand
Accurate demand forecasting is imperative for retailers to know how much each product they will need in the future. Sellers can predict future product demand by analyzing previous sales data and market trends. That helps them ensure they have enough of the suitable retail product datasets when needed so they don't end up with too many or too few things to sell.
What are the Types of Retail Analytics?
There are four main types of Retail data analytics. Diagnostic analytics helps find the cause of a problem; predictive analytics predicts future outcomes and prescriptive analytics suggests ways to fix issues. Descriptive analytics examines and explains past performance. Here's more detail about each of these methods.
Descriptive analytics
Descriptive analytics is the foundation for more advanced analytics. Basic analytics helps answer simple questions like "when, where, how many, and what." It creates essential business reports showing how much stock is available and sold weekly.
Diagnostic analytics
Retail stores can use diagnostic analytics to discover and solve problems affecting their success. By bringing together information from different places, such as how well they are doing financially, how well they are running, and what customers are saying, retail stores can better understand what is causing their challenges.
Predictive analytics
Retailers use special tools to predict what might happen in the future. They consider problems with getting products, the weather, how the economy is doing, and whether new stores are opening nearby. They might also consider what could happen if they do different things, like giving a slight discount compared to a significant discount. That helps them determine when they might run out of things to sell based on what might happen.
Prescriptive analytics
When AI and retail big data analysis work together, they can analyze information to suggest what to do next. That might include recommending products to customer service representatives based on what customers have bought before, like suggesting an upgrade or offering related products to help a new customer.
What is Retail Big Data Analysis?
Big data is essential for retail. Retailers use big data to understand market trends, customer behavior, and operational efficiency. They analyze large volumes of data from various sources, such as social media and transaction records. That helps them make better decisions about their products and how to run their stores more effectively.
What are the types of Retail Datasets?
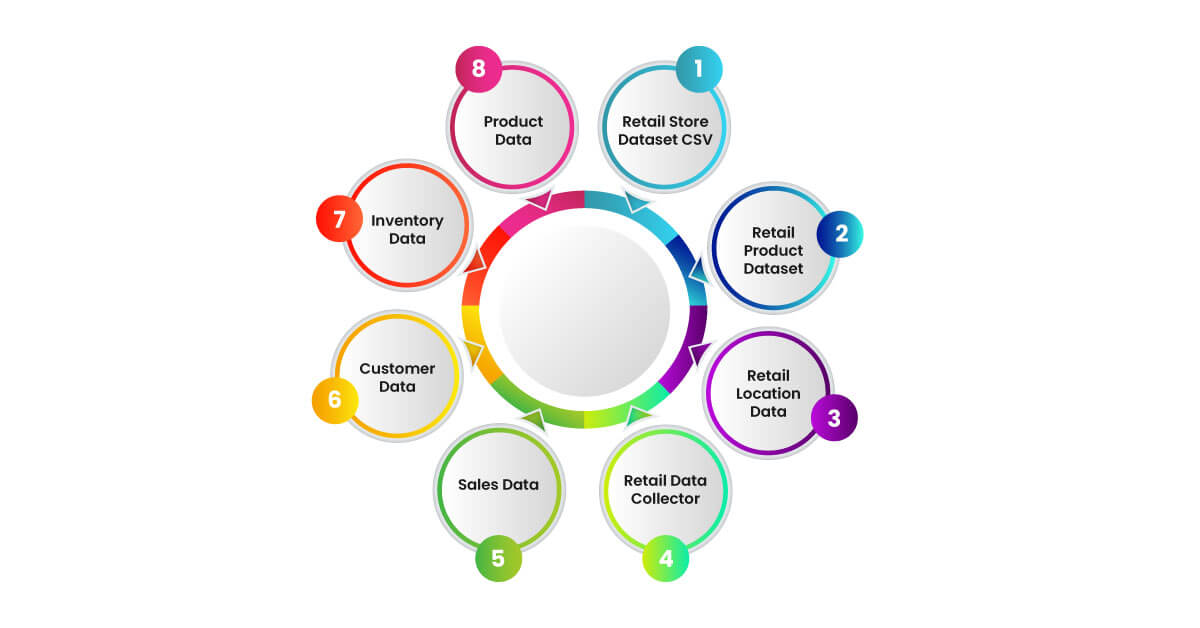
To analyze data effectively, retailers need to have access to relevant data. Retail datasets are collections of data related to various aspects of retail operations. Some common types of retail datasets include:
Retail Store Dataset CSV
This data details the things sold, how many are in stock, and how customers interact with the retail shops. It is usually kept in CSV format, which makes it simple to import into different software and examine.
Retail Product Dataset
A retail product dataset has information about the products a store sells, like what the products are, how much they cost, and how many are sold. This information is essential for understanding how well the products are doing and ensuring the store has the right amount of each product.
Retail Location Data
Retail location data includes store location, the number of people visiting them, and the amount of merchandise they sell in different areas. This information is essential for deciding where to put new stores, how to market products in other regions, and how to arrange the stores inside.
Retail Data Collector
A retail data collector is a tool or software that gathers information from cash registers, customer surveys, and online sales. This information is then kept in retail data sets to be studied later.
Sales Data
The sales data includes details about the products sold, the dates and times of the transactions, and the payment methods. This information is essential for analyzing sales trends, determining which products sell best, and understanding customers' preferences.
Customer Data
This data includes customers' age, where they live, what they have purchased before, and whether they are part of a particular customer program. Retailers study this data to group their customers, create personalized marketing, and improve their shopping experience.
Inventory Data
Inventory data is information about a store's stock, available products, and suppliers. Looking at this data helps retailers ensure that they have the right amount of stock, waste less, and better manage how products get from the suppliers to the store.
Product Data
Retail product datasets contain detailed product information, including descriptions, prices, categories, and supplier information. Retailers use this data to help with pricing, choosing which products to sell, and improving their displays.
What are the Challenges in Data Analytics for Retail?
While data analytics in retail offers many benefits, it also comes with challenges. Some of the most common challenges include:
- Data Quality: Ensuring that your information is correct is essential to getting the right results. Correcting or adding information can lead to better decisions. People who sell things need a way to check that their data is correct and clean it up regularly.
- Data Security: As retailers collect more information, keeping that information safe is essential. By using robust security, retailers must protect their customers and critical information from computer hackers.
- Integration of Data Sources: Retailers gather information from different sources, such as online shops, stores, and social media. Combining all this information can be challenging because it might be in various forms and systems. Retailers must use special tools and technology to combine all this information smoothly, which helps them see the big picture of their business's performance.
- Skilled Workforce: Understanding information from numbers and making decisions based on that information is essential for businesses. However, finding and keeping people with the right skills for this work can take time for retailers. Providing training and support for employees can help with this problem.
What are the Best Practices for Retail Data Analytics?
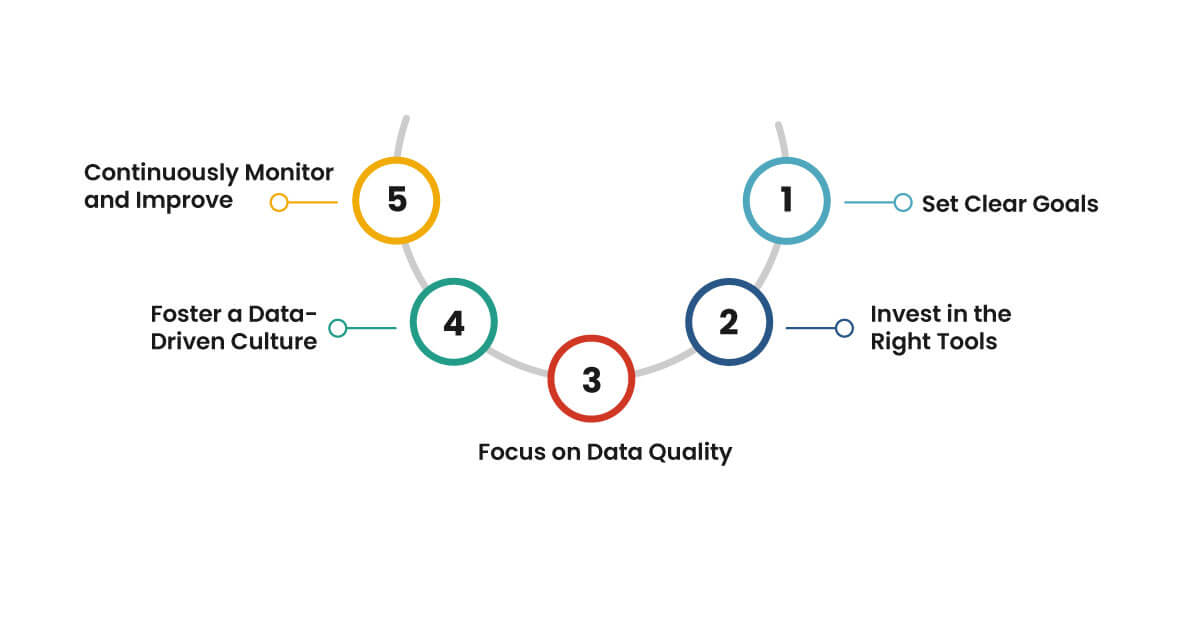
Businesses should follow these best practices to maximize the benefits of data analytics in the retail industry:
Set Clear Goals
Before starting a data analysis project, retailers should set clear goals and objectives. That will help with the analysis and ensure that the information learned matches the business's needs.
Invest in the Right Tools
Retailers should buy tools like big data platforms, AI and ML technologies, and data visualization tools to understand information better and gain helpful insights.
Focus on Data Quality
It's essential to ensure that the information is reliable so you can analyze the data accurately. In retail, this means regularly checking and cleaning the information and providing it from trustworthy sources.
Foster a Data-Driven Culture
To maximize data analysis in the retail industry, retailers should encourage employees to use data to make decisions and provide the proper training and tools.
Continuously Monitor and Improve
Retailers must always check their data analysis and improve it when necessary. That means keeping up with the newest technologies and trends in data analysis and regularly reviewing and enhancing their analysis plans.
Conclusion
Data analytics for retail helps retailers understand their business. It can improve sales, operations, and customer satisfaction. Data analysis in retail industry allows retailers to make better decisions and become more competitive. But to get the most out of it, they must deal with challenges like good data, security, and combining different systems.
Retailing has changed a lot because of data. We now use data from social media, online shopping, and smartphones to do business. That helps us make better decisions and understand our customers more. Using data can help us manage risks, perform better, and find important information. Because of these benefits, many retailers will keep using data to keep customers happy, improve their brand, and sell more. Data helps us learn about our customers and sell more to them. As technology becomes more important in retail, it's clear that using data is very important!
In today's changing retail world, using data analytics is no longer just a nice extra — it's something you need. Retailers who use data analytics and buy the right tools and technologies will be in an excellent position to succeed in the future. You can achieve success with Web Screen Scraping, which is your partner in data analytics solutions for retail.